Player FM - Internet Radio Done Right
Checked 16d ago
Menambahkan three tahun yang lalu
Konten disediakan oleh Klaviyo Data Science Team. Semua konten podcast termasuk episode, grafik, dan deskripsi podcast diunggah dan disediakan langsung oleh Klaviyo Data Science Team atau mitra platform podcast mereka. Jika Anda yakin seseorang menggunakan karya berhak cipta Anda tanpa izin, Anda dapat mengikuti proses yang diuraikan di sini https://id.player.fm/legal.
Player FM - Aplikasi Podcast
Offline dengan aplikasi Player FM !
Offline dengan aplikasi Player FM !
Podcast Layak Disimak
DISPONSORI
A
Action Academy | Replace The Job You Hate With A Life You Love


1 How To Replace A $100,000+ Salary Within 6 MONTHS Through Buying A Small Business w/ Alex Kamenca & Carley Mitus 57:50
57:50
Putar Nanti
Putar Nanti
Daftar
Suka
Menyukai57:50
Alex (@alex_kamenca) and Carley (@carleymitus) are both members of our Action Academy Community that purchased TWO small businesses last thursday! Want To Quit Your Job In The Next 6-18 Months Through Buying Commercial Real Estate & Small Businesses? 👔🏝️ Schedule A Free 15 Minute Coaching Call With Our Team Here To Get "Unstuck" Want to know which investment strategy is best for you? Take our Free Asset-Selection Quiz Check Out Our Bestselling Book : From Passive To Passionate : How To Quit Your Job - Grow Your Wealth - And Turn Your Passions Into Profits Want A Free $100k+ Side Hustle Guide ? Follow Me As I Travel & Build: IG @brianluebben ActionAcademy.com…
Klaviyo Data Science Podcast
Tandai semua (belum/sudah) diputar ...
Manage series 3251385
Konten disediakan oleh Klaviyo Data Science Team. Semua konten podcast termasuk episode, grafik, dan deskripsi podcast diunggah dan disediakan langsung oleh Klaviyo Data Science Team atau mitra platform podcast mereka. Jika Anda yakin seseorang menggunakan karya berhak cipta Anda tanpa izin, Anda dapat mengikuti proses yang diuraikan di sini https://id.player.fm/legal.
This podcast is intended for all audiences who love data science--veterans and newcomers alike, from any field, we’re all here to learn and grow our data science skills. New episodes monthly. Learn more about Klaviyo at www.klaviyo.com!
…
continue reading
58 episode
Tandai semua (belum/sudah) diputar ...
Manage series 3251385
Konten disediakan oleh Klaviyo Data Science Team. Semua konten podcast termasuk episode, grafik, dan deskripsi podcast diunggah dan disediakan langsung oleh Klaviyo Data Science Team atau mitra platform podcast mereka. Jika Anda yakin seseorang menggunakan karya berhak cipta Anda tanpa izin, Anda dapat mengikuti proses yang diuraikan di sini https://id.player.fm/legal.
This podcast is intended for all audiences who love data science--veterans and newcomers alike, from any field, we’re all here to learn and grow our data science skills. New episodes monthly. Learn more about Klaviyo at www.klaviyo.com!
…
continue reading
58 episode
Semua episode
×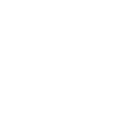
1 Klaviyo Data Science Podcast EP 58 | All Aboard the Leadership 48:22
48:22
Putar Nanti
Putar Nanti
Daftar
Suka
Menyukai48:22
All successful teams have at least one leader, and most have at least one manager. This episode, we dive into how leadership works on highly technical teams, how managing a highly technical team works, and why the two aren’t exactly the same thing. Listen along for more discussion about: The traits of highly effective leaders — and how that might look different on an engineering or data science team How to know that a move into management fits you Our guests’ best recommendations for management books, resources, and experiences For more details, including links to the many resources our panel suggest to learn more about leadership and management, check out the full writeup on Medium !…
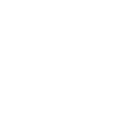
1 Klaviyo Data Science Podcast EP 57 | Agile, or, Don't Go Chasing Waterfall 44:53
44:53
Putar Nanti
Putar Nanti
Daftar
Suka
Menyukai44:53
What is agile methodology — and, just as importantly, what is it not? Whether you’re new to agile entirely or you stay up late pondering its most philosophical inner workings, if you want to know more about agile and how organizations can reap its benefits while avoiding its pitfalls, this is the episode for you. You’ll learn about a variety of topics, including: How to effectively compromise between the tenets of agile and the realities of building software — and how not to When agile helps you pivot, and what that means for your customers Our guests’ hottest takes about agile For the full show notes, including who's who, see the Medium writeup .…
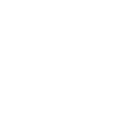
1 Klaviyo Data Science Podcast EP 56 | Evaluating AI Models: A Seminar (feat. Evan Miller) 45:29
45:29
Putar Nanti
Putar Nanti
Daftar
Suka
Menyukai45:29
This month, the Klaviyo Data Science Podcast welcomes Evan Miller to deliver a seminar on his recently published paper, Adding Error Bars to Evals: A Statistical Approach to Language Model Evaluations ! This episode is a mix of a live seminar Evan gave to the team at Klaviyo and an interview we conducted with him afterward. Suppose you’re trying to understand the performance of an AI model — maybe one you built or fine-tuned and are comparing to state-of-the-art models, maybe one you’re considering loading up and using for a project you’re about to start. If you look at the literature today, you can get a sense of what the average performance for the model is on an evaluation or set of tasks. But often, that’s unfortunately the extent of what it’s possible to learn —there is much less emphasis placed on the variability or uncertainty inherent to those estimates. And as anyone who’s worked with a statistical model in the past can affirm, variability is a huge part of why you might choose to use or discard a model. This seminar explores how to best compute, summarize, and display estimates of variability for AI models. Listen along to hear about topics like: Why the Central Limit Theorem you learned about in Stats 101 is still relevant with the most advanced AI models developed today How to think about complications of classic assumptions, such as measurement error or clustering, in the AI landscape When to do a sample size calculation for your AI model, and how to do it About Evan Miller You may already know our guest Evan Miller from his fantastic blog , which includes his celebrated A/B testing posts, such as “How not to run an A/B test.” You may also have used his A/B testing tools , such as the sample size calculator. Evan currently works as a research scientist at Anthropic. About Anthropic Per Anthropic’s website : You can find more information about Anthropic, including links to their social media accounts, on the company website. Anthropic is an AI safety and research company based in San Francisco. Our interdisciplinary team has experience across ML, physics, policy, and product. Together, we generate research and create reliable, beneficial AI systems. Special thanks to Chris Murphy at Klaviyo for organizing this seminar and making this episode possible! For the full show notes, including who's who, see the Medium writeup .…
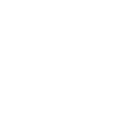
1 Klaviyo Data Science Podcast EP 55 | 2024 Year in Review 49:55
49:55
Putar Nanti
Putar Nanti
Daftar
Suka
Menyukai49:55
Welcome back to the Klaviyo Data Science podcast! This episode, we dive into… 2024 Year in Review As the new year starts, we take a look back at 2024. We spoke to data scientists and people who work closely with data scientists, and we asked them all the question we ask every year: what is the coolest data science thing you learned about in 2024? You’ll hear a wide range of answers, including: How a rhyme can topple LLM security Why the Sequential Probably Ratio Test is better at measuring basketball ability than the NBA playoffs How badly a non-specialized LLM could beat you at chess For the full show notes, including who's who, see the Medium writeup .…
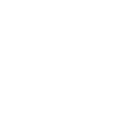
1 Klaviyo Data Science Podcast EP 54| The Right to Exclude 41:13
41:13
Putar Nanti
Putar Nanti
Daftar
Suka
Menyukai41:13
How we protect invention and ingenuity: Patents Writing software often involves taking known patterns, combining and shaping them, and adding needed context or specialization related to the problem we’re trying to solve. Sometimes, that means writing something that’s effectively been written by someone else before. But sometimes, that means creating something new. What should you do in a case where you’ve genuinely created something new? Perhaps more importantly, how do you know when you’re in that situation? This month, we explore one of the best tools to help answer both questions: the patent process. Listen along with your fearless co-hosts and a member of Klaviyo’s legal team to learn about what a patent is, why getting them matters, and how to get your own novel work patented, along with: Tips to keep in mind when applying for a patent Knowing which software you should try to patent How to get your organization to buy into patents For the full show notes, including who's who, see the Medium writeup .…
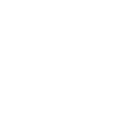
1 Klaviyo Data Science Podcast EP 53 | Yahoogle 48:21
48:21
Putar Nanti
Putar Nanti
Daftar
Suka
Menyukai48:21
Welcome to the November episode of the Klaviyo Data Science Podcast for 2024! In years past, November episodes reflected the chaotic Black Friday/Cyber Monday season by examining unique challenges of readiness, scale, and fundamental changes happening with little to no warning, as well as how those challenges were handled; this November is no different. What happens when two of the largest email platforms make sweeping changes to their spam filters, providing a few short months of notice? Stress, uncertainty, and an opportunity for individuals and organization to rise to the challenge. In this month’s episode, we talk with analysts, engineers, and product managers to discuss Klaviyo’s journey to meet Yahoo and Google’s new Email Delivery Requirements — aka Yahoogle, the colloqial name for a new set of rules that must be followed by email senders to have their emails make it to inboxes and not go straight to the junk bin. Listen in to hear more about: Yahoogle. It’s more than just a funny word! The challenges in operating a large-scale email sending system Advice and retrospectives about large, cross-functional projects and tight deadlines For the full show notes, including who's who, see the Medium writeup .…
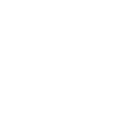
1 Klaviyo Data Science Podcast EP 52 | Our Favorite Models and How They Work 51:08
51:08
Putar Nanti
Putar Nanti
Daftar
Suka
Menyukai51:08
As most data scientists will tell you, there is no such thing as the single best model or the perfect model . Some work well in some circumstances but poorly in others, some present a specific tradeoff between factors like flexibility and explainability that is only useful in certain settings. Some are best set up to handle specific types of data that don’t arise in every single project. But at the same time, most data scientists would acknowledge that some models manage to stand out. Maybe it’s nostalgia, maybe it’s how powerful they are in some settings, maybe it’s another factor entirely — but for one reason or another, most data scientists will admit they have a soft spot for some models. That’s what we’re here to discuss this month: what is your favorite model? Listen in to hear more about: The benefits of simple models How learning about some models teaches you about entire fields of mathematics What fitting a classification model has to teach us about epistemology For the full show notes, including who's who, see the Medium writeup .…
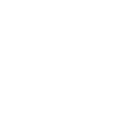
1 Klaviyo Data Science Podcast EP 51 | How Personalization Empowers Users 44:10
44:10
Putar Nanti
Putar Nanti
Daftar
Suka
Menyukai44:10
If you’re making software, especially data science-powered software, there’s a good chance one of your biggest goals is to empower stronger and deeper personalization for your users. Our topic for this month: how can you do even more than that? How can we make personalization not just robust, but both more effective and easier than the alternative? It’s not a simple task, but it is one that the team we interviewed this month has tackled. Listen in to hear more about: Why personalization is a matter of finishing your user’s… sandwiches How to approach complex personalization features as a data scientist, a designer, or an engineer What the game of Battleship can teach you about personalization For the full show notes, including who's who, see the Medium writeup .…
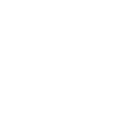
1 Klaviyo Data Science Podcast EP 50 | The 50th Episode Celebration Special 44:40
44:40
Putar Nanti
Putar Nanti
Daftar
Suka
Menyukai44:40
It may come as a suprise to those of you reading this, but this milestone snuck up on me. I was surprised to realize we’d reached a full 50 episodes. What better time to take a moment to reflect and look back? This episode is all about the Klaviyo Data Science Podcast. We talk through the history of the podcast, how we approach making episodes that matter to our listeners, our highlight episodes, and what we’ve learned through the years. You’ll hear about: Why you don’t need a fancy mic to get started How to approach talking about deep, technical work on the air What we have planned for the next 50 episodes For the full show notes, including who's who, see the Medium writeup .…
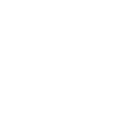
1 Klaviyo Data Science Podcast EP 49 | What Real Data Scientists Wish They'd Known Earlier in their Careers 43:30
43:30
Putar Nanti
Putar Nanti
Daftar
Suka
Menyukai43:30
A big part of growing and developing as a data scientist, or any other member of a data science team, is taking time to reflect, learn, and distill experiences into advice. This month, we’ve asked four senior members of the data science team to do exactly that: look back over their careers, reflect on what they know and what they wished they’d known earlier, and tell everyone what those lessons are. Listen to this advice-filled episode to hear: How taking a more scenic or indirect career route can impart valuable experiences The growth and development opportunities that truly unlocked new phases of their careers, and how you can make the most of similar opportunities Why mistakes often make the best learning opportunities For the full show notes, including who's who, see the Medium writeup .…
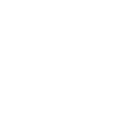
1 Klaviyo Data Science Podcast EP 48 | Data Science Goes Worldwide 42:36
42:36
Putar Nanti
Putar Nanti
Daftar
Suka
Menyukai42:36
Internationalizing your product There are many aspects of product growth — reaching new heights for peak volume, reaching new levels of sustained daily volume, growing your feature set and the complexity of your code based, and many others. Dealing with growth in an intelligent and forward-looking way is never easy, but this month we deal with a type of growth that presents its own unique set of challenges: international growth, i.e. expanding the range of countries and languages your products are natively available in. This month, we talked with multiple members of the internationalization effort here at Klaviyo, from teams across our organization. You’ll hear about: How strings can be far more complex than they seem Why changing languages doesn’t just mean changing words Where in the world your assumptions about language may break For the full show notes, including who's who, see the Medium writeup .…
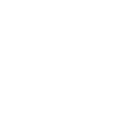
1 Klaviyo Data Science Podcast EP 47 | Cooking Up Something Special with Data Science: Made In Cookware 48:10
48:10
Putar Nanti
Putar Nanti
Daftar
Suka
Menyukai48:10
How real marketers use data science We spend a lot of time on this podcast talking about how to build data science solutions. Implicit in many of those conversations is perhaps the most fundamental truth of product design and development: we build data science solutions because people use them. We aren’t doing this just for fun — the reason we spend so much time, effort, and energy to refine our solutions is that it actually matters to real people. This month, we talk to some of those people. In particular, we sat down with two members of the team at Made In Cookware ( http://madeincookware.com/ ) to discuss what makes their business unique, how they approach understanding and marketing to their customers, and how data science and AI help them do all of that. You’ll hear about: What kitchen knives can teach you about product design and development Which type of pan you should use to cook a steak, and how that can help you understand customer segmentation How AI saves real marketers real time while also giving them better results About Made In Made In Cookware (Made In) is a premium cookware brand based in Austin, TX. Founded in 2017 but born of a 4th-generation, family-owned kitchen supply business, Made In creates best-in-class cookware developed in partnership with the world’s finest chefs and foremost craftsmen. Today, you’ll find Made In products in more than 2,000 restaurants, in the hands of James Beard Award-winning chefs at Michelin-starred restaurants across the country, and in the kitchens of home cooks everywhere. Made In products have garnered over 100,000 5-star reviews, and the company was named one of Inc. Magazine’s best workplaces and Newsweek’s best online shops of 2024. For the full show notes, including who's who, see the Medium writeup .…
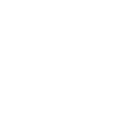
1 Klaviyo Data Science Podcast EP 46 | ML Ops 101 45:10
45:10
Putar Nanti
Putar Nanti
Daftar
Suka
Menyukai45:10
An Introduction to ML Ops Building data science products requires many things we’ve discussed on this podcast before: insight, customer empathy, strategic thinking, flexibility, and a whole lot of determination. But it requires one more thing we haven’t talked about nearly as much: a stable, performant, and easy-to-use foundation. Setting up that foundation is the chief goal of the field of machine learning operations, aka ML Ops. This month on the Klaviyo Data Science Podcast, we give a brief but thorough introduction to the field of ML Ops. You’ll hear about: How ML Ops is different from the similar fields of data science and DevOps What skills a successful ML Ops developer should have, and what an ML Ops developer’s day-to-day looks like Why concepts like “velocity” and “stability” have their own special nuances in the world of ML Ops For the full show notes, including who's who, see the Medium writeup .…
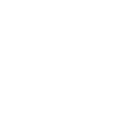
1 Klaviyo Data Science Podcast EP 45 | SegmentsAI: An AI Case Study on Delivering Value 42:14
42:14
Putar Nanti
Putar Nanti
Daftar
Suka
Menyukai42:14
In many ways, 2023 was the year of AI in tech, which is a double-edged sword. On the one hand, the basic technology is straightforwardly exciting — but on the other hand, with seemingly every technology solution scrambling to integrate a thin wrapper around ChatGPT, it’s hard to stand out in a saturated environment. This month on the Klaviyo Data Science Podcast, we dive into a case study of how to build AI products, SegmentsAI, and discuss the principles that go into making sure your AI-powered product shines — and, more importantly, actually helps your customers. You’ll hear about: How to know when AI is the right solution for the problem The unique technical challenges that come with building an AI product, from user testing to validation The answer to the AI chicken-and-egg problem “Why do this, why build another LLM feature? It seems like every website is rushing to get their name next to AI... How you break through the noise is to actually provide value to people, not novelty. Being able to help customers speed up or generate new, interesting segments that they otherwise wouldn’t? I think that’s valuable.”— Rob Huselid, Senior Data Scientist For the full show notes, including who's who, see the Medium writeup .…
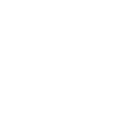
1 Klaviyo Data Science Podcast EP 44 | The Data Powering EDI 52:17
52:17
Putar Nanti
Putar Nanti
Daftar
Suka
Menyukai52:17
Welcome back to the Klaviyo Data Science podcast! This episode, we dive into… Equity, Diversity, and Inclusion Equity, diversity, and inclusion (EDI) are more than just central principles of successful teams in data science and beyond — they’re also a rich field that presents interesting and challenging data science problems. This episode, we chat with two EDI specialists at Klaviyo about EDI, the data that powers it, and the challenges that come with using that data. You’ll hear about: Why EDI is a core part of both processes and products How to work with self-reported data — and, sometimes, work around the fact that you don’t actually have the data you want Examples of EDI work in action For the full show notes, including who's who, see the Medium writeup .…
K
Klaviyo Data Science Podcast

1 Klaviyo Data Science Podcast EP 43 | 2023: A Data Science Year in Review 1:56:23
1:56:23
Putar Nanti
Putar Nanti
Daftar
Suka
Menyukai1:56:23
2023 Year in Review As the new year starts, we take a look back at 2023. We spoke to 11 data scientist and people who work closely with data scientists, and we asked them all the question we ask every year: what is the coolest data science thing you learned about in 2023? You’ll hear a wide range of answers, including: How data science moving to peripheral devices and becoming more accessible has huge implications for the future of the field Peculiarities of working with large language models, both in terms of the tasks they can carry out and how the process of working with them is more complicated than it seems at first How powerful simple techniques can be at even highly complex tasks “You don’t have to have a PhD any longer to do data science. And I think that’s amazing and powerful, and it’s going to mean that the future is… where everybody is allowed to do data science stuff without having lots and lots of education.” — Wayne Coburn, Director, Product Management For the full show notes, including stories mentioned in the episode and who's who, see the Medium writeup .…
K
Klaviyo Data Science Podcast

1 Klaviyo Data Science Podcast EP 42 | Unlocking Customer Insights with RFM 40:04
40:04
Putar Nanti
Putar Nanti
Daftar
Suka
Menyukai40:04
Welcome back to the Klaviyo Data Science podcast! This episode, we dive into… Knowing your customers Customers are all unique, whether you’re building a data science product or selling an ecommerce product. In an ideal world, we’d be able to think about all of them on a truly one-on-one basis. Most of us can’t keep track of that many people in our brains, though, which is where the topic of today’s episode comes in: what is the best way to summarize an entire population of customers into a number of groups that is small enough to intuit but fine-grained enough to actually be useful in practice? Listen along to learn more about: Why understanding your customers is the superpower that drives any successful product How simple-sounding concepts like recency can be trickier than expected How to build a technical solution that draws on a vast number of data stores For the full show notes, including resources mentioned in the episode and who's who, see the Medium writeup .…
K
Klaviyo Data Science Podcast

1 Klaviyo Data Science Podcast EP 41 | Incident Response, or: How I Learned to Stop Worrying and Break Production 46:50
46:50
Putar Nanti
Putar Nanti
Daftar
Suka
Menyukai46:50
Welcome back to the Klaviyo Data Science podcast! This episode, we dive into… When Things Break Welcome to the November episode of the Klaviyo Data Science Podcast for this year! November is a unique month for ecommerce, which makes it a unique month for any software solution built for ecommerce; it’s a tradition on this podcast to take the opportunity to celebrate some of those unique challenges. In an ideal world, software and data science products would never break. We do not live in an ideal world, though, so an important question to answer is: what should you do when things do break? This month, we discuss incidents, incident response, and getting things back on track as quickly and effectively as possible to continue delivering value to your customers. Listen along to learn more about: Why not all ways of recognizing something has gone wrong are created equal How to cut through disagreements when the stakes are at their highest What sorts of unique challenges data science breakages and incidents pose For the full show notes, including resources mentioned in the episode and who's who, see the Medium writeup .…
K
Klaviyo Data Science Podcast

1 Klaviyo Data Science Podcast EP 40 | Platform Abuse and Misuse 43:30
43:30
Putar Nanti
Putar Nanti
Daftar
Suka
Menyukai43:30
Welcome back to the Klaviyo Data Science podcast! This episode, we dive into… Off the Happy Path In most discussions about data science and data science features on this podcast, we make a basic, foundational assumption: the users whose data we are thinking about and customer experience we are trying to improve are, generally speaking, trying to use the platform in a way we recognize and approve of. Not all users of an application have this intention, and the data science behind detecting users who misuse a platform— and even abuse it — constitutes a complex and vast field of study. Listen along to learn more about: Different types of human behaviors motivating platform misuse, and how that translates to different types of data What makes many-to-one problems so challenging Why keywords alone are not enough For the full show notes, including who's who, see the Medium writeup .…
K
Klaviyo Data Science Podcast

1 Klaviyo Data Science Podcast EP 39 | Are you going to science fair? 1:07:15
1:07:15
Putar Nanti
Putar Nanti
Daftar
Suka
Menyukai1:07:15
Welcome back to the Klaviyo Data Science podcast! This episode, we dive into… Presenting your work for fun and profit Presenting technical work is not something you automatically learn how to do — just like the technical skills themselves, it has to be learned and practiced, and opportunities to practice it can be hard to find. This episode, we discuss one opportunity that Klaviyo put together for its R&D teams this summer: the Klaviyo R&D Science Fair. Listen along to hear about: How, much like software development, explaining technical work is an iterative process The best ways to engage a crowd and get them interested in what you have to say The unique and powerful allure of scissors and glue guns “We put together a little game: try to find all of the accessibility problems in this form, without using the tool that we built…. And then when they react, ‘oh my God, like that one was impossible, I don’t know how you expected me to find that,’ that’s when we can say: exactly! That’s why we needed this feature!”— Maya Nigrin, Senior Software Engineer For the full show notes, including photos of the event, see the Medium writeup .…
K
Klaviyo Data Science Podcast

1 Klaviyo Data Science Podcast EP 38 | Production 101 42:01
42:01
Putar Nanti
Putar Nanti
Daftar
Suka
Menyukai42:01
Welcome back to the Klaviyo Data Science podcast! This episode, we dive into… An introduction to production What comes after you finish building a data science model? If you’re working on a software project, the answer likely involves that model serving customers in production. Understanding production is crucial for any data scientist or software engineer, so we spend this episode learning about best practices from three experienced Klaviyo engineers. Listen along to learn more about: How to make sure your code is “battle-ready,” whether you’re working on a data science project or not Why error messages you think are safe to ignore may not actually be safe to ignore One key lesson for safely deploying your code, no matter what environment you work in “That’s stuck with me through the years: there are these knock-on effects between things. Even if it’s not your code, you should still try to understand how it’s working and whether it can have a ripple effect that comes back and affects your code.”— Chris Conlon, Lead Software Engineer Check out the full show notes on Medium !…
K
Klaviyo Data Science Podcast

1 Klaviyo Data Science Podcast EP 37 | How research works (part 1) 46:12
46:12
Putar Nanti
Putar Nanti
Daftar
Suka
Menyukai46:12
Welcome back to the Klaviyo Data Science podcast! This episode, we dive into… Research is a core part of data science. But data science is far from alone in that respect — other fields rely on research just as heavily, and they have their own set of hypotheses, methods, complications, and concerns. This month, we talk to three Klaviyos about research they did before joining the team — both data science research and other kinds — to see what we can learn about conducting effective data science research. Listen along to learn more about: What tiny iron meteorites teach us about the importance of using your results to tell a compelling story What data science research into commerce and policy teaches us about iterating on your research questions What rubber beams teach us about the importance of getting feedback early “Everybody has a unique perspective could be the one that opens up a brand new door. You’re looking at doing specific algorithms, you’re looking at doing the research a specific way, but there could be an alternative path.” - Mike Galli, Data Scientist See the full writeup on Medium !…
K
Klaviyo Data Science Podcast

1 Klaviyo Data Science Podcast EP 36 | There's No Place Like Home (Page) 42:09
42:09
Putar Nanti
Putar Nanti
Daftar
Suka
Menyukai42:09
Welcome back to the Klaviyo Data Science podcast! This episode, we dive into… Few parts of your product, application, or webpage are more crucial than the very initial experience. In a web application like Klaviyo, that means the home page. Everyone sees it every time they log on to do anything, and interactions with that page set the tone for everything that follows. Meaning: if you’re going to change the home page, you need to really know what you’re doing. This month, we talk with the Klaviyo engineering team that did just that. We discuss many aspects of that redesign, including: How to get buy-in from teams you depend on without taking away your own independence The unique difficulties that come with large front-end engineering projects and smart data visualization How to filter through the noise when evaluating the success of a feature “There are very few features ever been released in Klaviyo that have seen that sort of change… At the end of the day, if we can help our users complete tasks faster and more effectively, that’s our highest priority.” - Griffin Drigotas, Senior Product Designer See the full writeup on Medium !…
K
Klaviyo Data Science Podcast

1 Klaviyo Data Science Podcast EP 35 | How to become a data scientist 39:42
39:42
Putar Nanti
Putar Nanti
Daftar
Suka
Menyukai39:42
Welcome back to the Klaviyo Data Science podcast! This episode, we dive into… The question is slightly tongue-in-cheek, but only slightly. Data science is a new field — while many people today are graduating with degrees in data science, the same was not true a decade ago. Many of the people who work (and will work) as data scientists were not classically trained as a data scientist, but as something else. This month, we examine that process: the process of working in a field that’s distinct from data science and becoming a data scientist. We discuss several parts of that journey, including: What attracts someone to data science in the first place How to approach gaining the technical skills you need to get a data science job How similar some parts of the data scientist job are to washing dishes Where do data scientists come from?“You really need to practice using these tools. I did my best to come up with excuses to use data science techniques in all my projects… maybe instead of trying to automate a workflow in Excel VBA, I’d try to automate it in python instead.” - Steven Her, Data Scientist Read the full writeup on Medium !…
K
Klaviyo Data Science Podcast

1 Klaviyo Data Science Podcast EP 34 | Books every data scientist should read (vol. 3) 44:19
44:19
Putar Nanti
Putar Nanti
Daftar
Suka
Menyukai44:19
Welcome back to the Klaviyo Data Science podcast! This episode, we dive into… Back by popular demand: data science is a broad, deep field with an extraordinary amount to learn, and we’re here to help you learn it. We asked four members of the Data Science team at Klaviyo what one of their favorite data science books was, and we got four different answers. Listen on if you’ve wanted to know more ways to learn about: How to think about and employ the Bayesian framework (and corgis) Learning intro-to-intermediate coding skills necessary for data science work The theory that drives natural language processing The mindset of a data scientist in general “it gives you a different lens to apply to different problems. And sometimes taking that different lens, suddenly a problem that was really hard to formulate using traditional frequentist statistics or machine learning techniques, suddenly it can be really easy to frame in this other way” - Tommy Blanchard, Senior Data Science Manager Read the full writeup on Medium !…
K
Klaviyo Data Science Podcast

1 Klaviyo Data Science Podcast EP 33 | How to found a (data science) team 57:38
57:38
Putar Nanti
Putar Nanti
Daftar
Suka
Menyukai57:38
Listen to the full episode on Anchor , or in your favorite podcast distribution platform! Welcome back to the Klaviyo Data Science podcast! This episode, we dive into… Starting from scratch We’ve talked about a lot of aspects of data science on this podcast — building software features, conducting research, learning new methods and skills, recruiting new members — but there’s one we’ve always avoided: building a new team from the ground up. A large reason for that is personnel — while your cohosts may be intrepid, they are not experts in this area. This month, we bring on two people who are: Eric Silberstein and Ezra Freedman, who founded the Data Science team at Klaviyo. We draw on their wealth of experience, knowledge, and lessons learned the hard way while founding a young team. As you might expect, these lessons extend beyond data science teams in particular — whether you’re founding another team or starting a new business, or looking to join a team in its early stages, you might be able to learn from our discussions, such as: How setting concrete goals is key for a new team How to think about your first hire, and your next five How to steer a team through large organizational changes while maintaining its culture and essence “When you view the world, do you think of it as ‘if-then’ statements, or do you tend to think of it as some sort of function to optimize? Our team needed both.” - Eric Silberstein, VP of Data Science Read the full writeup on Medium !…
K
Klaviyo Data Science Podcast

1 Klaviyo Data Science Podcast EP 32 | How iOS 15 changed the world (and data science answered) 39:46
39:46
Putar Nanti
Putar Nanti
Daftar
Suka
Menyukai39:46
Welcome back to the Klaviyo Data Science podcast! This episode, we dive into… When the data science world changes When you work in data science, it’s inevitable that the world will change for you. Sometimes it’s due to global events, macroeconomic trends, or sudden shifts in consumer behavior. Other times it’s due to new features added by a commonly-used piece of software. When your lifeblood is data, all of these can be equally shocking and disruptive. This month, we discuss one of the latter cases: the changes to the world of email marketing data brought about by the iOS 15 privacy updates. We bring on a panel of product managers, data scientists, and software engineers to discuss: How one software update can drastically alter your data landscape How to do research while the world is changing, and how to test your conclusions while the ground truth is still in flux How using different sources of data can help you adapt your product to a new reality “That was the biggest thing I came out of this with. Being first doesn’t really matter if what you’re delivering isn’t the right solution for your customers”— Nader Fotouhi, Lead Product Manager Read the full writeup on Medium !…
K
Klaviyo Data Science Podcast

1 Klaviyo Data Science Podcast EP 31 | 2022: A Data Science Year in Review 1:06:09
1:06:09
Putar Nanti
Putar Nanti
Daftar
Suka
Menyukai1:06:09
Welcome back to the Klaviyo Data Science podcast! This episode, we dive into… 2022 Year in Review As the new year starts, we take a look back at 2022. We spoke to 8 data scientist and people who work closely with data scientists, and we asked them all the same question: what is the coolest data science thing you learned about in 2022? You’ll hear about fascinating data science topics, including: Advances in AI, NLP, and data science in general in 2022 How understanding data science and ML operations makes a recruiter’s job easier New ways to classify models, visualize data, and play video games “I think these models have been really good for a long while. It’s a snowball effect of people realizing they’ve been good and seeing how much cool stuff can be built with them.” - Robert Huselid, Data Scientist Read the full writeup on Medium !…
K
Klaviyo Data Science Podcast

1 Klaviyo Data Science Podcast EP 30 | These Are a Few of our Favorite Tools 51:39
51:39
Putar Nanti
Putar Nanti
Daftar
Suka
Menyukai51:39
Welcome back to the Klaviyo Data Science podcast! This episode, we dive into… Tools of the Trade We talk a lot on this podcast about the results of data science and software engineering work. We even talk about the process of doing data science and software engineering work. But one thing we haven’t shed much light on, until this month, is: what specific tools help a Data Science team — or any developer or data scientist similarly engaged in building a scalable and intelligent system — actually do their work? We asked several data scientists, machine learning engineers, software engineers, designers, and product managers the same question: what is your favorite tool that helps you do your job? You’ll hear all their answers in this episode, including: Why some well-known tools fully deserve the hype Specialized packages for specialized purposes How to slow down and really force yourself to think about the problem How to avoid analysis paralysis “I like banging out unstyled web forms as much as the next back-end developer, but when you have the experience of spending all day in a tool, those ‘tiny’ things like icon consistency really pay off.” — Zac Bentley, Lead Site Reliability Engineer II Read the full show notes, meet this month's guests, and learn more about Klaviyo in our Medium writeup !…
K
Klaviyo Data Science Podcast

1 Klaviyo Data Science Podcast EP 29 | Detecting the Unexpected 42:59
42:59
Putar Nanti
Putar Nanti
Daftar
Suka
Menyukai42:59
Welcome back to the Klaviyo Data Science podcast! This episode, we dive into… Anomaly Detection It’s our third November on the Klaviyo Data Science Podcast, and if you work in ecommerce, you know that November means one thing: Black Friday and (usually) Cyber Monday, i.e. the month of the year where everything changes. Traditionally, we’ve talked about things that help prepare builders of software for when the world is about to change, such as infrastructure, readiness, scale-out testing, and other things along those lines. This year, we’re approaching it from another angle: ecommerce stores go through the exact same struggle every year. How can a platform like Klaviyo help prepare them for the unexpected? One answer: by automatically figuring out when unexpected things are happening, i.e., by detecting anomalous behavior. You’ll hear all about anomaly detection on this episode, including: How to pivot your research when your idea is valuable but your results aren’t providing that value How to label large swaths of data efficiently How to design algorithms for an extraordinarily diverse base of end users “Imagine a sneaker company who does product drops compared to a regular ecommerce brand. Then imagine customers who are just starting up, with very low traffic…. It was definitely a challenge to generalize to the entire Klaviyo customer base.” — Harsh Mehta, Senior Machine Learning Engineer Read the full show notes on Medium !…
Selamat datang di Player FM!
Player FM memindai web untuk mencari podcast berkualitas tinggi untuk Anda nikmati saat ini. Ini adalah aplikasi podcast terbaik dan bekerja untuk Android, iPhone, dan web. Daftar untuk menyinkronkan langganan di seluruh perangkat.