Join Token Metrics Podcast, hosted by Ian Balina, for sharp crypto market analysis, the latest news, and trend forecasting. Your go-to for keeping abreast with the dynamic crypto world.
…
continue reading
Discover potential 100x opportunities with Crypto Hidden Gems. We spotlight early-stage crypto projects, serving as your guide to future crypto titans before they surge.
…
continue reading
Start your crypto journey with our Crypto Investing Guide. Arm yourself with strategic tips and tools for confident and savvy crypto investing.
…
continue reading
Time is money, and with Crypto Minute, we make every second count. Dive into crisp 15-60 second updates and insights on crypto trading and investing, keeping you in the loop with the ever-evolving crypto space.
…
continue reading
XRPodcast is a new platform to discuss developments within the XRP ecosystem, digital asset and crypto communities. We will frequently conduct interviews with digital asset and crypto leaders. Support this podcast: https://podcasters.spotify.com/pod/show/podcastxrp/support
…
continue reading
This podcast talks about how to program in Java; not your tipical system.out.println("Hello world"), but more like real issues, such as O/R setups, threading, getting certain components on the screen or troubleshooting tips and tricks in general. The format is as a podcast so that you can subscribe to it, and then take it with you and listen to it on your way to work (or on your way home), and learn a little bit more (or reinforce what you knew) from it.
…
continue reading
Running out of time to catch up with new arXiv papers? We take the most impactful papers and present them as convenient podcasts. If you're a visual learner, we offer these papers in an engaging video format. Our service fills the gap between overly brief paper summaries and time-consuming full paper reads. You gain academic insights in a time-efficient, digestible format. Code behind this work: https://github.com/imelnyk/ArxivPapers Support this podcast: https://podcasters.spotify.com/pod/s ...
…
continue reading
The world’s 2nd largest cryptocurrency Ethereum is going through an upgrade! It’s previous problems will now be solved with Eth 2.0. In this podcast series, Christine Kim and Ben Edgington, CoinDesks’ Eth 2.0 Dream Team, talk about the live development of Ethereum 2.0, as it phases through technical hurdles and upgrades from proof of work to proof of stake. Join the conversation as Christine and Ben, spotlight the major news events related to Eth 2.0 and walk us through its potential impact ...
…
continue reading
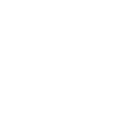
1
[QA] Active-Dormant Attention Heads: Mechanistically Demystifying Extreme-Token Phenomena in LLMs
7:44
7:44
Putar nanti
Putar nanti
Daftar
Suka
Menyukai
7:44
The paper investigates extreme-token phenomena in transformer-based LLMs, revealing mechanisms behind attention sinks and proposing strategies to mitigate their impact during pretraining. https://arxiv.org/abs//2410.13835 YouTube: https://www.youtube.com/@ArxivPapers TikTok: https://www.tiktok.com/@arxiv_papers Apple Podcasts: https://podcasts.appl…
…
continue reading
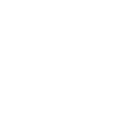
1
Active-Dormant Attention Heads: Mechanistically Demystifying Extreme-Token Phenomena in LLMs
17:44
17:44
Putar nanti
Putar nanti
Daftar
Suka
Menyukai
17:44
The paper investigates extreme-token phenomena in transformer-based LLMs, revealing mechanisms behind attention sinks and proposing strategies to mitigate their impact during pretraining. https://arxiv.org/abs//2410.13835 YouTube: https://www.youtube.com/@ArxivPapers TikTok: https://www.tiktok.com/@arxiv_papers Apple Podcasts: https://podcasts.appl…
…
continue reading
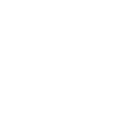
1
[QA] MOVIE GEN: A Cast of Media Foundation Models
8:52
8:52
Putar nanti
Putar nanti
Daftar
Suka
Menyukai
8:52
https://arxiv.org/abs//2410.13720 YouTube: https://www.youtube.com/@ArxivPapers TikTok: https://www.tiktok.com/@arxiv_papers Apple Podcasts: https://podcasts.apple.com/us/podcast/arxiv-papers/id1692476016 Spotify: https://podcasters.spotify.com/pod/show/arxiv-papers --- Support this podcast: https://podcasters.spotify.com/pod/show/arxiv-papers/supp…
…
continue reading
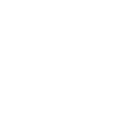
1
MOVIE GEN: A Cast of Media Foundation Models
1:53:06
1:53:06
Putar nanti
Putar nanti
Daftar
Suka
Menyukai
1:53:06
https://arxiv.org/abs//2410.13720 YouTube: https://www.youtube.com/@ArxivPapers TikTok: https://www.tiktok.com/@arxiv_papers Apple Podcasts: https://podcasts.apple.com/us/podcast/arxiv-papers/id1692476016 Spotify: https://podcasters.spotify.com/pod/show/arxiv-papers --- Support this podcast: https://podcasters.spotify.com/pod/show/arxiv-papers/supp…
…
continue reading
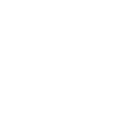
1
[QA] One Step Diffusion via Shortcut Models
8:06
8:06
Putar nanti
Putar nanti
Daftar
Suka
Menyukai
8:06
https://arxiv.org/abs//2410.12557 YouTube: https://www.youtube.com/@ArxivPapers TikTok: https://www.tiktok.com/@arxiv_papers Apple Podcasts: https://podcasts.apple.com/us/podcast/arxiv-papers/id1692476016 Spotify: https://podcasters.spotify.com/pod/show/arxiv-papers --- Support this podcast: https://podcasters.spotify.com/pod/show/arxiv-papers/supp…
…
continue reading
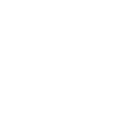
1
One Step Diffusion via Shortcut Models
17:39
17:39
Putar nanti
Putar nanti
Daftar
Suka
Menyukai
17:39
https://arxiv.org/abs//2410.12557 YouTube: https://www.youtube.com/@ArxivPapers TikTok: https://www.tiktok.com/@arxiv_papers Apple Podcasts: https://podcasts.apple.com/us/podcast/arxiv-papers/id1692476016 Spotify: https://podcasters.spotify.com/pod/show/arxiv-papers --- Support this podcast: https://podcasters.spotify.com/pod/show/arxiv-papers/supp…
…
continue reading
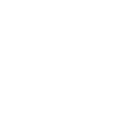
1
[QA] Inference Scaling for Long-Context Retrieval Augmented Generation
7:17
7:17
Putar nanti
Putar nanti
Daftar
Suka
Menyukai
7:17
https://arxiv.org/abs//2410.04343 YouTube: https://www.youtube.com/@ArxivPapers TikTok: https://www.tiktok.com/@arxiv_papers Apple Podcasts: https://podcasts.apple.com/us/podcast/arxiv-papers/id1692476016 Spotify: https://podcasters.spotify.com/pod/show/arxiv-papers --- Support this podcast: https://podcasters.spotify.com/pod/show/arxiv-papers/supp…
…
continue reading
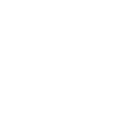
1
Inference Scaling for Long-Context Retrieval Augmented Generation
22:02
22:02
Putar nanti
Putar nanti
Daftar
Suka
Menyukai
22:02
https://arxiv.org/abs//2410.04343 YouTube: https://www.youtube.com/@ArxivPapers TikTok: https://www.tiktok.com/@arxiv_papers Apple Podcasts: https://podcasts.apple.com/us/podcast/arxiv-papers/id1692476016 Spotify: https://podcasters.spotify.com/pod/show/arxiv-papers --- Support this podcast: https://podcasters.spotify.com/pod/show/arxiv-papers/supp…
…
continue reading
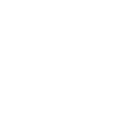
1
[QA] What Matters in Transformers? Not All Attention is Needed
8:07
8:07
Putar nanti
Putar nanti
Daftar
Suka
Menyukai
8:07
This study explores redundancy in Transformer architectures, revealing that many attention layers can be pruned with minimal performance loss, enhancing efficiency for large language models. https://arxiv.org/abs//2406.15786 YouTube: https://www.youtube.com/@ArxivPapers TikTok: https://www.tiktok.com/@arxiv_papers Apple Podcasts: https://podcasts.a…
…
continue reading
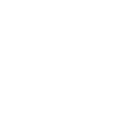
1
What Matters in Transformers? Not All Attention is Needed
16:20
16:20
Putar nanti
Putar nanti
Daftar
Suka
Menyukai
16:20
This study explores redundancy in Transformer architectures, revealing that many attention layers can be pruned with minimal performance loss, enhancing efficiency for large language models. https://arxiv.org/abs//2406.15786 YouTube: https://www.youtube.com/@ArxivPapers TikTok: https://www.tiktok.com/@arxiv_papers Apple Podcasts: https://podcasts.a…
…
continue reading
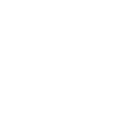
1
[QA] Language Models Encode Numbers Using Digit Representations in Base 10
7:33
7:33
Putar nanti
Putar nanti
Daftar
Suka
Menyukai
7:33
The paper investigates how large language models represent numbers, revealing they use digit-wise circular representations, which explains their frequent errors in numerical reasoning tasks. https://arxiv.org/abs//2410.11781 YouTube: https://www.youtube.com/@ArxivPapers TikTok: https://www.tiktok.com/@arxiv_papers Apple Podcasts: https://podcasts.a…
…
continue reading
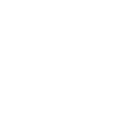
1
Language Models Encode Numbers Using Digit Representations in Base 10
10:36
10:36
Putar nanti
Putar nanti
Daftar
Suka
Menyukai
10:36
The paper investigates how large language models represent numbers, revealing they use digit-wise circular representations, which explains their frequent errors in numerical reasoning tasks. https://arxiv.org/abs//2410.11781 YouTube: https://www.youtube.com/@ArxivPapers TikTok: https://www.tiktok.com/@arxiv_papers Apple Podcasts: https://podcasts.a…
…
continue reading
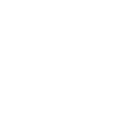
1
[QA] Don't Transform the Code, Code the Transforms: Towards Precise Code Rewriting using LLMs
7:31
7:31
Putar nanti
Putar nanti
Daftar
Suka
Menyukai
7:31
This paper explores using large language models to generate code transformations through a chain-of-thought approach, demonstrating improved precision and adaptability compared to direct code rewriting methods. https://arxiv.org/abs//2410.08806 YouTube: https://www.youtube.com/@ArxivPapers TikTok: https://www.tiktok.com/@arxiv_papers Apple Podcasts…
…
continue reading
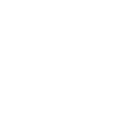
1
Don't Transform the Code, Code the Transforms: Towards Precise Code Rewriting using LLMs
6:15
6:15
Putar nanti
Putar nanti
Daftar
Suka
Menyukai
6:15
This paper explores using large language models to generate code transformations through a chain-of-thought approach, demonstrating improved precision and adaptability compared to direct code rewriting methods. https://arxiv.org/abs//2410.08806 YouTube: https://www.youtube.com/@ArxivPapers TikTok: https://www.tiktok.com/@arxiv_papers Apple Podcasts…
…
continue reading
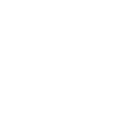
1
[QA] Do Unlearning Methods Remove Information from Language Model Weights?
8:03
8:03
Putar nanti
Putar nanti
Daftar
Suka
Menyukai
8:03
The paper evaluates unlearning techniques in Large Language Models, revealing that current methods inadequately remove sensitive information, allowing attackers to recover significant pre-unlearning accuracy. https://arxiv.org/abs//2410.08827 YouTube: https://www.youtube.com/@ArxivPapers TikTok: https://www.tiktok.com/@arxiv_papers Apple Podcasts: …
…
continue reading
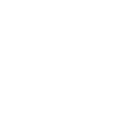
1
Do Unlearning Methods Remove Information from Language Model Weights?
17:56
17:56
Putar nanti
Putar nanti
Daftar
Suka
Menyukai
17:56
The paper evaluates unlearning techniques in Large Language Models, revealing that current methods inadequately remove sensitive information, allowing attackers to recover significant pre-unlearning accuracy. https://arxiv.org/abs//2410.08827 YouTube: https://www.youtube.com/@ArxivPapers TikTok: https://www.tiktok.com/@arxiv_papers Apple Podcasts: …
…
continue reading
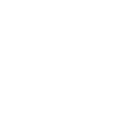
1
[QA] MLE-bench:Â Evaluating Machine Learning Agents on Machine Learning Engineering
7:19
7:19
Putar nanti
Putar nanti
Daftar
Suka
Menyukai
7:19
MLE-bench is a benchmark for evaluating AI agents in machine learning engineering, featuring 75 Kaggle competitions and establishing human baselines, with open-source code for future research. https://arxiv.org/abs//2410.07095 YouTube: https://www.youtube.com/@ArxivPapers TikTok: https://www.tiktok.com/@arxiv_papers Apple Podcasts: https://podcasts…
…
continue reading
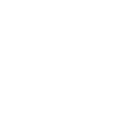
1
MLE-bench:Â Evaluating Machine Learning Agents on Machine Learning Engineering
16:41
16:41
Putar nanti
Putar nanti
Daftar
Suka
Menyukai
16:41
MLE-bench is a benchmark for evaluating AI agents in machine learning engineering, featuring 75 Kaggle competitions and establishing human baselines, with open-source code for future research. https://arxiv.org/abs//2410.07095 YouTube: https://www.youtube.com/@ArxivPapers TikTok: https://www.tiktok.com/@arxiv_papers Apple Podcasts: https://podcasts…
…
continue reading
https://arxiv.org/abs//2410.07073 YouTube: https://www.youtube.com/@ArxivPapers TikTok: https://www.tiktok.com/@arxiv_papers Apple Podcasts: https://podcasts.apple.com/us/podcast/arxiv-papers/id1692476016 Spotify: https://podcasters.spotify.com/pod/show/arxiv-papers --- Support this podcast: https://podcasters.spotify.com/pod/show/arxiv-papers/supp…
…
continue reading
https://arxiv.org/abs//2410.07073 YouTube: https://www.youtube.com/@ArxivPapers TikTok: https://www.tiktok.com/@arxiv_papers Apple Podcasts: https://podcasts.apple.com/us/podcast/arxiv-papers/id1692476016 Spotify: https://podcasters.spotify.com/pod/show/arxiv-papers --- Support this podcast: https://podcasters.spotify.com/pod/show/arxiv-papers/supp…
…
continue reading
DIFF Transformer enhances attention to relevant context while reducing noise, improving performance in language modeling, long-context tasks, and in-context learning, making it a promising architecture for large language models. https://arxiv.org/abs//2410.05258 YouTube: https://www.youtube.com/@ArxivPapers TikTok: https://www.tiktok.com/@arxiv_pap…
…
continue reading
DIFF Transformer enhances attention to relevant context while reducing noise, improving performance in language modeling, long-context tasks, and in-context learning, making it a promising architecture for large language models. https://arxiv.org/abs//2410.05258 YouTube: https://www.youtube.com/@ArxivPapers TikTok: https://www.tiktok.com/@arxiv_pap…
…
continue reading
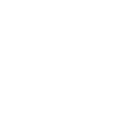
1
[QA] GSM-Symbolic: Understanding the Limitations of Mathematical Reasoning in Large Language Models
7:45
7:45
Putar nanti
Putar nanti
Daftar
Suka
Menyukai
7:45
This study introduces GSM-Symbolic, a benchmark revealing LLMs' inconsistent mathematical reasoning, highlighting performance drops with altered questions and increased complexity, questioning their genuine logical reasoning abilities. https://arxiv.org/abs//2410.05229 YouTube: https://www.youtube.com/@ArxivPapers TikTok: https://www.tiktok.com/@ar…
…
continue reading
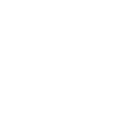
1
GSM-Symbolic: Understanding the Limitations of Mathematical Reasoning in Large Language Models
12:39
12:39
Putar nanti
Putar nanti
Daftar
Suka
Menyukai
12:39
This study introduces GSM-Symbolic, a benchmark revealing LLMs' inconsistent mathematical reasoning, highlighting performance drops with altered questions and increased complexity, questioning their genuine logical reasoning abilities. https://arxiv.org/abs//2410.05229 YouTube: https://www.youtube.com/@ArxivPapers TikTok: https://www.tiktok.com/@ar…
…
continue reading
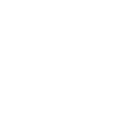
1
[QA] Efficient Dictionary Learning with Switch Sparse Autoencoders
7:43
7:43
Putar nanti
Putar nanti
Daftar
Suka
Menyukai
7:43
Switch Sparse Autoencoders efficiently scale feature extraction in neural networks by routing activations through smaller expert models, improving reconstruction and sparsity while reducing computational costs. https://arxiv.org/abs//2410.08201 YouTube: https://www.youtube.com/@ArxivPapers TikTok: https://www.tiktok.com/@arxiv_papers Apple Podcasts…
…
continue reading
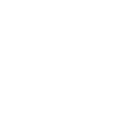
1
Efficient Dictionary Learning with Switch Sparse Autoencoders
15:44
15:44
Putar nanti
Putar nanti
Daftar
Suka
Menyukai
15:44
Switch Sparse Autoencoders efficiently scale feature extraction in neural networks by routing activations through smaller expert models, improving reconstruction and sparsity while reducing computational costs. https://arxiv.org/abs//2410.08201 YouTube: https://www.youtube.com/@ArxivPapers TikTok: https://www.tiktok.com/@arxiv_papers Apple Podcasts…
…
continue reading
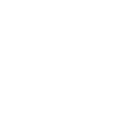
1
[QA] Visual Scratchpads: Enabling Global Reasoning in Vision
7:22
7:22
Putar nanti
Putar nanti
Daftar
Suka
Menyukai
7:22
This paper introduces global visual benchmarks, highlighting modern vision models' struggles with global reasoning and proposing 'visual scratchpads' to enhance learning efficiency and generalization. https://arxiv.org/abs//2410.08165 YouTube: https://www.youtube.com/@ArxivPapers TikTok: https://www.tiktok.com/@arxiv_papers Apple Podcasts: https://…
…
continue reading
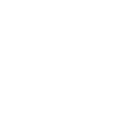
1
Visual Scratchpads: Enabling Global Reasoning in Vision
26:26
26:26
Putar nanti
Putar nanti
Daftar
Suka
Menyukai
26:26
This paper introduces global visual benchmarks, highlighting modern vision models' struggles with global reasoning and proposing 'visual scratchpads' to enhance learning efficiency and generalization. https://arxiv.org/abs//2410.08165 YouTube: https://www.youtube.com/@ArxivPapers TikTok: https://www.tiktok.com/@arxiv_papers Apple Podcasts: https://…
…
continue reading
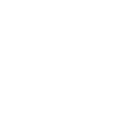
1
[QA] RL, but don't do anything I wouldn't do
7:43
7:43
Putar nanti
Putar nanti
Daftar
Suka
Menyukai
7:43
The paper critiques KL regularization in reinforcement learning, showing it fails with Bayesian predictive models, and proposes a new principle to better control advanced RL agent behavior. https://arxiv.org/abs//2410.06213 YouTube: https://www.youtube.com/@ArxivPapers TikTok: https://www.tiktok.com/@arxiv_papers Apple Podcasts: https://podcasts.ap…
…
continue reading
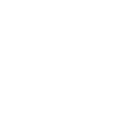
1
RL, but don't do anything I wouldn't do
16:19
16:19
Putar nanti
Putar nanti
Daftar
Suka
Menyukai
16:19
The paper critiques KL regularization in reinforcement learning, showing it fails with Bayesian predictive models, and proposes a new principle to better control advanced RL agent behavior. https://arxiv.org/abs//2410.06213 YouTube: https://www.youtube.com/@ArxivPapers TikTok: https://www.tiktok.com/@arxiv_papers Apple Podcasts: https://podcasts.ap…
…
continue reading
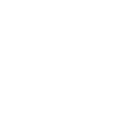
1
[QA] Restructuring Vector Quantization with the Rotation Trick
7:43
7:43
Putar nanti
Putar nanti
Daftar
Suka
Menyukai
7:43
This paper proposes a method to propagate gradients through VQ-VAEs' vector quantization layer, improving reconstruction metrics, codebook utilization, and quantization error across various training paradigms. https://arxiv.org/abs//2410.06424 YouTube: https://www.youtube.com/@ArxivPapers TikTok: https://www.tiktok.com/@arxiv_papers Apple Podcasts:…
…
continue reading
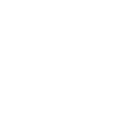
1
Restructuring Vector Quantization with the Rotation Trick
23:23
23:23
Putar nanti
Putar nanti
Daftar
Suka
Menyukai
23:23
This paper proposes a method to propagate gradients through VQ-VAEs' vector quantization layer, improving reconstruction metrics, codebook utilization, and quantization error across various training paradigms. https://arxiv.org/abs//2410.06424 YouTube: https://www.youtube.com/@ArxivPapers TikTok: https://www.tiktok.com/@arxiv_papers Apple Podcasts:…
…
continue reading
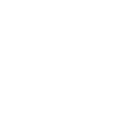
1
[QA] EnsemW2S: Can an Ensemble of LLMs be Leveraged to Obtain a Stronger LLM?
7:42
7:42
Putar nanti
Putar nanti
Daftar
Suka
Menyukai
7:42
This research proposes an innovative ensemble method for weak-to-strong generalization in AI, enhancing LLM performance through collaborative supervision, achieving significant improvements on challenging tasks. https://arxiv.org/abs//2410.04571 YouTube: https://www.youtube.com/@ArxivPapers TikTok: https://www.tiktok.com/@arxiv_papers Apple Podcast…
…
continue reading
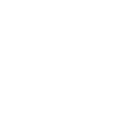
1
EnsemW2S: Can an Ensemble of LLMs be Leveraged to Obtain a Stronger LLM?
18:39
18:39
Putar nanti
Putar nanti
Daftar
Suka
Menyukai
18:39
This research proposes an innovative ensemble method for weak-to-strong generalization in AI, enhancing LLM performance through collaborative supervision, achieving significant improvements on challenging tasks. https://arxiv.org/abs//2410.04571 YouTube: https://www.youtube.com/@ArxivPapers TikTok: https://www.tiktok.com/@arxiv_papers Apple Podcast…
…
continue reading
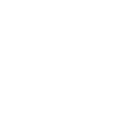
1
[QA] Density estimation with LLMs: a geometric investigation of in-context learning trajectories
8:13
8:13
Putar nanti
Putar nanti
Daftar
Suka
Menyukai
8:13
This study explores LLaMA-2's in-context learning for probability density estimation, revealing unique learning trajectories and interpreting its behavior as adaptive kernel density estimation. https://arxiv.org/abs//2410.05218 YouTube: https://www.youtube.com/@ArxivPapers TikTok: https://www.tiktok.com/@arxiv_papers Apple Podcasts: https://podcast…
…
continue reading
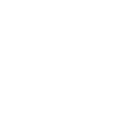
1
Density estimation with LLMs: a geometric investigation of in-context learning trajectories
12:31
12:31
Putar nanti
Putar nanti
Daftar
Suka
Menyukai
12:31
This study explores LLaMA-2's in-context learning for probability density estimation, revealing unique learning trajectories and interpreting its behavior as adaptive kernel density estimation. https://arxiv.org/abs//2410.05218 YouTube: https://www.youtube.com/@ArxivPapers TikTok: https://www.tiktok.com/@arxiv_papers Apple Podcasts: https://podcast…
…
continue reading
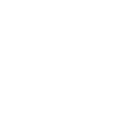
1
[QA] Teaching Transformers Modular Arithmetic at Scale
8:29
8:29
Putar nanti
Putar nanti
Daftar
Suka
Menyukai
8:29
This paper enhances modular addition in machine learning by introducing diverse training data, angular embedding, and a custom loss function, improving performance for cryptographic applications and other modular arithmetic problems. https://arxiv.org/abs//2410.03569 YouTube: https://www.youtube.com/@ArxivPapers TikTok: https://www.tiktok.com/@arxi…
…
continue reading
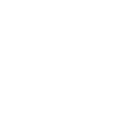
1
Teaching Transformers Modular Arithmetic at Scale
13:01
13:01
Putar nanti
Putar nanti
Daftar
Suka
Menyukai
13:01
This paper enhances modular addition in machine learning by introducing diverse training data, angular embedding, and a custom loss function, improving performance for cryptographic applications and other modular arithmetic problems. https://arxiv.org/abs//2410.03569 YouTube: https://www.youtube.com/@ArxivPapers TikTok: https://www.tiktok.com/@arxi…
…
continue reading
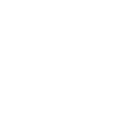
1
[QA] What Matters for Model Merging at Scale?
7:57
7:57
Putar nanti
Putar nanti
Daftar
Suka
Menyukai
7:57
This study evaluates model merging at scale, revealing insights on expert model quality, size, and merging methods, ultimately enhancing generalization and performance in large-scale applications. https://arxiv.org/abs//2410.03617 YouTube: https://www.youtube.com/@ArxivPapers TikTok: https://www.tiktok.com/@arxiv_papers Apple Podcasts: https://podc…
…
continue reading
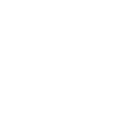
1
What Matters for Model Merging at Scale?
24:32
24:32
Putar nanti
Putar nanti
Daftar
Suka
Menyukai
24:32
This study evaluates model merging at scale, revealing insights on expert model quality, size, and merging methods, ultimately enhancing generalization and performance in large-scale applications. https://arxiv.org/abs//2410.03617 YouTube: https://www.youtube.com/@ArxivPapers TikTok: https://www.tiktok.com/@arxiv_papers Apple Podcasts: https://podc…
…
continue reading
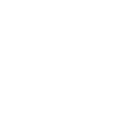
1
[QA] Depth Pro: Sharp Monocular Metric Depth in Less Than a Second
7:17
7:17
Putar nanti
Putar nanti
Daftar
Suka
Menyukai
7:17
Depth Pro is a fast foundation model for zero-shot monocular depth estimation, producing high-resolution, metric depth maps without metadata, outperforming previous methods in accuracy and detail. https://arxiv.org/abs//2410.02073 YouTube: https://www.youtube.com/@ArxivPapers TikTok: https://www.tiktok.com/@arxiv_papers Apple Podcasts: https://podc…
…
continue reading
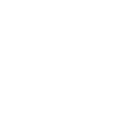
1
Depth Pro: Sharp Monocular Metric Depth in Less Than a Second
14:08
14:08
Putar nanti
Putar nanti
Daftar
Suka
Menyukai
14:08
Depth Pro is a fast foundation model for zero-shot monocular depth estimation, producing high-resolution, metric depth maps without metadata, outperforming previous methods in accuracy and detail. https://arxiv.org/abs//2410.02073 YouTube: https://www.youtube.com/@ArxivPapers TikTok: https://www.tiktok.com/@arxiv_papers Apple Podcasts: https://podc…
…
continue reading
This work revisits LSTMs and GRUs, introducing minimal versions that eliminate hidden state dependencies, enabling efficient parallel training while matching the performance of recent sequence models. https://arxiv.org/abs//2410.01201 YouTube: https://www.youtube.com/@ArxivPapers TikTok: https://www.tiktok.com/@arxiv_papers Apple Podcasts: https://…
…
continue reading
This work revisits LSTMs and GRUs, introducing minimal versions that eliminate hidden state dependencies, enabling efficient parallel training while matching the performance of recent sequence models. https://arxiv.org/abs//2410.01201 YouTube: https://www.youtube.com/@ArxivPapers TikTok: https://www.tiktok.com/@arxiv_papers Apple Podcasts: https://…
…
continue reading
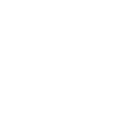
1
[QA] OOD-CHAMELEON: Is Algorithm Selection for OOD Generalization Learnable?
8:08
8:08
Putar nanti
Putar nanti
Daftar
Suka
Menyukai
8:08
The paper introduces OOD-CHAMELEON, a method for selecting algorithms for out-of-distribution generalization by predicting performance based on dataset characteristics, outperforming individual algorithms and heuristics. https://arxiv.org/abs//2410.02735 YouTube: https://www.youtube.com/@ArxivPapers TikTok: https://www.tiktok.com/@arxiv_papers Appl…
…
continue reading
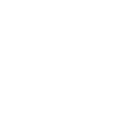
1
OOD-CHAMELEON: Is Algorithm Selection for OOD Generalization Learnable?
21:51
21:51
Putar nanti
Putar nanti
Daftar
Suka
Menyukai
21:51
The paper introduces OOD-CHAMELEON, a method for selecting algorithms for out-of-distribution generalization by predicting performance based on dataset characteristics, outperforming individual algorithms and heuristics. https://arxiv.org/abs//2410.02735 YouTube: https://www.youtube.com/@ArxivPapers TikTok: https://www.tiktok.com/@arxiv_papers Appl…
…
continue reading
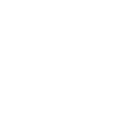
1
[QA] Training Language Models on Synthetic Edit Sequences Improves Code Synthesis
7:53
7:53
Putar nanti
Putar nanti
Daftar
Suka
Menyukai
7:53
The paper presents LintSeq, a synthetic data generation algorithm that refactors code into edit sequences, improving LLM performance in code synthesis and achieving state-of-the-art results with smaller models. https://arxiv.org/abs//2410.02749 YouTube: https://www.youtube.com/@ArxivPapers TikTok: https://www.tiktok.com/@arxiv_papers Apple Podcasts…
…
continue reading
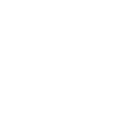
1
Training Language Models on Synthetic Edit Sequences Improves Code Synthesis
18:46
18:46
Putar nanti
Putar nanti
Daftar
Suka
Menyukai
18:46
The paper presents LintSeq, a synthetic data generation algorithm that refactors code into edit sequences, improving LLM performance in code synthesis and achieving state-of-the-art results with smaller models. https://arxiv.org/abs//2410.02749 YouTube: https://www.youtube.com/@ArxivPapers TikTok: https://www.tiktok.com/@arxiv_papers Apple Podcasts…
…
continue reading
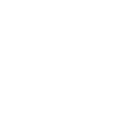
1
[QA] Automated Red Teaming with GOAT: the Generative Offensive Agent Tester
7:19
7:19
Putar nanti
Putar nanti
Daftar
Suka
Menyukai
7:19
https://arxiv.org/abs//2410.01606 YouTube: https://www.youtube.com/@ArxivPapers TikTok: https://www.tiktok.com/@arxiv_papers Apple Podcasts: https://podcasts.apple.com/us/podcast/arxiv-papers/id1692476016 Spotify: https://podcasters.spotify.com/pod/show/arxiv-papers --- Support this podcast: https://podcasters.spotify.com/pod/show/arxiv-papers/supp…
…
continue reading
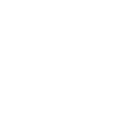
1
Automated Red Teaming with GOAT: the Generative Offensive Agent Tester
13:38
13:38
Putar nanti
Putar nanti
Daftar
Suka
Menyukai
13:38
https://arxiv.org/abs//2410.01606 YouTube: https://www.youtube.com/@ArxivPapers TikTok: https://www.tiktok.com/@arxiv_papers Apple Podcasts: https://podcasts.apple.com/us/podcast/arxiv-papers/id1692476016 Spotify: https://podcasters.spotify.com/pod/show/arxiv-papers --- Support this podcast: https://podcasters.spotify.com/pod/show/arxiv-papers/supp…
…
continue reading